From the Desk of Gus Mueller
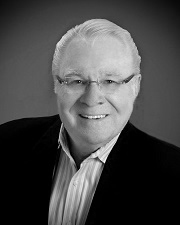
Today, it seems that the implementation of artificial intelligence (AI) is just about everywhere. On your drive home, Google Maps suggests you take an alternative route, which will save you 10 minutes. AI? Sure. On Saturday evening, you casually Google “kayaks,” and on Sunday morning, you have an ad from L.L.Bean in your personal Facebook account. AI at work? Most certainly. A regular sausage eater from North Dakota has his Visa card declined in a Southern California Safeway when trying to buy kale. Result of AI? Could be!
What about hearing aids? It’s been nearly 20 years since we’ve experienced the emergence of hearing aids with trainable gain, which quickly advanced to trainable compression and then situation-specific training. But a lot has happened since then. So much so that it’s well worth devoting this month’s 20Q to a review of what AI-enhanced hearing aid features currently are available, and what is on the horizon. And who better to write about this than Josh Alexander, PhD, one of the leading experts on making sense of hearing aid technology.
Dr. Alexander is an Associate Professor in the Department of Speech, Language, and Hearing Sciences at Purdue University, where he has recently been appointed the Innovation and Entrepreneurship Fellow for the College of Health and Human Sciences. You probably know him for his publications regarding hearing aid technology, and the sophisticated hearing aid simulator that he has developed, which replicates the features of commercial hearing aids. He also is part of a team that recently opened the Accessible Precision Audiology Research Center in the 16 Tech Innovation District near Purdue University in Indianapolis, designed to enhance research collaborations with other universities and medical industries and focus on innovation surrounding health equity within the urban community.
Dr. Alexander certainly is no stranger to AudiologyOnline. Not only is this his 4th visit to our 20Q page, but his many text courses and webinars cover a wide range of hearing-aid related topics, with total offerings of 20 CEUs or more!
So . . . what AI things really are happening with hearing aids these days? How smart are they? And, is there really a “HatGPT?” You can find the answers to all this and more in Josh’s comprehensive review.
Gus Mueller, PhD
Contributing Editor
Browse the complete collection of 20Q with Gus Mueller CEU articles at www.audiologyonline.com/20Q
20Q: Artificial Intelligence in Hearing Aids - HatGPT
Learning Outcomes
After reading this article, professionals will be able to:
- Identify the key advancements in AI technology that have been integrated into modern hearing aids.
- Explain how AI algorithms in hearing aids adapt to users’ listening environments and preferences.
- Describe the role of sensors and AI in enhancing hearing aid functionality.
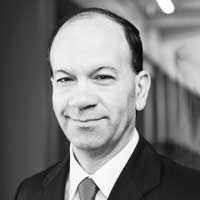
1. I’ve heard a lot about AI in the news lately and thought I was sort of keeping up, but what the heck is HatGPT?
Well, I am not too surprised you have not heard of it yet since it’s actually an acronym I just made up to catch your attention! I am capitalizing on all the media hype around artificial intelligence (AI) ever since OpenAI first introduced ChatGPT to the public at the end of 2022. So, what is HatGPT? It stands for “Hearing Aid Technology Guided by Predictive Techniques,” and I think you will find it perfectly captures the cutting-edge essence of hearing aid technology today (Figure 1). Just like ChatGPT revolutionized how we interact with AI, HatGPT is set to transform how we think about and use hearing aids. Buckle up because it will be a fun and fascinating ride into the future of hearing aids!
Figure 1. The integration of artificial intelligence (AI) into hearing aids is transforming the way we think about and use these devices. This image depicts an imaginary hearing aid designed to read brain activity, illustrating cutting-edge AI-driven advancements in hearing aid technology, whimsically dubbed “Hearing Aid Technology Guided by Predictive Techniques” (HatGPT). The essence behind HatGPT is to personalize and enhance user experiences by leveraging AI to adapt to individual needs and environmental contexts. As AI continues to evolve, its role in hearing aids will become increasingly vital, offering unprecedented levels of customization and user satisfaction.
As you know, AI has transformed numerous industries in recent years by introducing automation, personalization, and advanced data analysis. Before we get too deep into our discussion, let us take a few steps back.
Artificial Intelligence is a relatively broad term encompassing many methods and processes, including “machine learning” and its subfields, such as Bayesian optimization and “deep (neural network) learning.” Others have educated us about differences (e.g., Bramsløw and Beck, 2021; Andersen et al., 2021; Fabry and Browmik, 2021); however, for the average user, these distinctions blur into a broad concept called “AI.” While AI has been around relatively long, it has recently become incredibly accessible, requiring only a few simple keystrokes or voice commands. Such accessibility has eliminated the need for advanced programming skills or complex inputs, making sophisticated capabilities widely available to the general public. Today’s experience with AI feels distinctly different; it is more tangible and within reach than ever. This progress in accessible AI has been nothing short of breathtaking — absolutely breathtaking. It is reminiscent of the major technological revolutions of the 80s and 90s with the advent of affordable personal computers and the internet. Now, we can engage with digital assistants in ways once imagined only in futuristic cartoon figures like George Jetson, marking the onset of what feels like the next major technological revolution.
Figure 2. ChatGPT rendition of ‘George Jetson interacting with a robot.’
You heard it here first: “Accessible AI” is the term I have coined for what we are currently experiencing with tools like Open AI’s ChatGPT, Google’s Genesis, and many more. It is only in its infancy; for example, Figure 3 shows hilarious attempts to get ChatGPT (DALL-E) to draw a simple picture of a hearing aid. It can only get better from here!
Figure 3. From left to right, results from ChatGPT when prompted to draw a hearing aid.
While not bad, the first attempt leaves a little to be desired as it appears to be a mini-BTE with traditional earmold tubing, but it lacks the earpiece. The second attempt resulted from a prompt to have it draw a “receiver in the canal (RIC) hearing aid.” I am not sure what all the buttons could be used for or how the typical user might have the fine finger dexterity to manipulate all of them, and the earpiece looks like nothing I would ever send my patient home with as a permanent solution. Giving up on getting any sensible results, the third prompt was to draw a picture of a “behind the ear (BTE) hearing aid.” I have no words! Finally, I asked ChatGPT to draw a “typical hearing aid user,” resulting in the fourth image. Admittedly, the ability to draw human faces is truly remarkable; however, the thing in his ear looks less like a hearing aid and more like the Bluetooth earpieces that ‘really important’ people walked around with in the mid-2000s for hands-free calling.
2. How might AI enhance the functionality and user experience of hearing aids in the future?
Increased computing power, knowledge, and awareness have allowed AI to permeate many aspects of our everyday lives; the hearing aid industry is no exception. Believe it or not, AI has been instrumental in hearing aids for years, perhaps starting with the 1999 release of the NAL-NL1 (the first version of the National Acoustics Laboratory fitting prescription for Nonlinear amplification). A computer optimization scheme, a primitive version of AI, was used to derive gain-frequency responses for speech presented at 11 different input levels to 52 different audiogram configurations (Dillon, 1999). The basic goal was to maximize speech audibility using a modified model of the Speech Intelligibility Index (SII) while using a psychoacoustic model to keep the loudness of the amplified speech less than or equal to what would be experienced by an individual with normal hearing. Another AI model was then used to generate one equation (the NAL-NL1 fitting prescription) that summarized the optimizations for all 572 (or 11 times 52) gain–frequency responses.
3. That is impressive! What about the use of AI in hearing aids?
First, it is worth noting that users have interacted with AI in hearing aids for a long time. Following the initial introduction of AI in hearing aids nearly two decades ago, the technology continued to evolve. As noted by Taylor and Mueller (2023), early models focused on learning a user’s preferred volume through adjustments, and this adaptation happened quite quickly within a week or two. Interestingly, this personalization occurred in real-world situations rather than needing controlled clinical settings. However, these initial systems had limitations. For instance, volume adjustments for loud sounds could unintentionally affect softer ones. This hurdle was addressed by introducing more sophisticated AI that could not only learn overall volume preferences but also adapt to different sound levels and frequencies (Chalupper et al., 2009). This advancement allowed for better fine-tuning based on specific listening environments.
Getting to answer your original question, Figure 4 represents a hypothetical representation incorporating many ways AI is already implemented in hearing aids. AI is used in the research and development (R&D) phase to train on datasets and create new algorithms that go into the hearing aid as automatic features. The automatic features use AI-trained models to customize and personalize the hearing aid parameters based on the predicted acoustic scene. Imagine sound processing algorithms as tools with knobs and dials. These knobs and dials are the parameters, which are basically numbers the hearing aid can adjust. They control how the algorithm works on a sound. The more parameters an algorithm has, the more knobs and dials it has, allowing for finer control. This is handy in noisy places like bars and restaurants (Crow et al., 2021). By tweaking these parameters, the hearing aid can fine-tune the tool algorithm to better handle the specific challenges of that environment, resulting in better performance.
Because users’ listening intent, needs, or preferences may vary from the automatic solution, users can interact with the hearing aid in one form or another using AI decision-making methods to ‘teach’ the automatics and refine its parameter selection (e.g., Jensen et al., 2023). This information can then be fed to the ‘cloud’ (not an ethereal concept, as the name implies, but a tangible network of physical data servers) for analysis. By using AI to aggregate data from numerous users, manufacturers may be able to refine their features for a user’s listening goal in a specific situation. This iterative process can help hearing aid technology become smarter and more responsive with each generation or firmware update (Jensen et al., 2023), like how consumer feedback is used to continually improve products in other tech industries.
Figure 4. Illustration of the cyclical integration of AI in hearing aids, from its role in research and development (R&D) of automatic features through user interaction to cloud-based data analysis, resulting in a continuous enhancement of hearing aid functionality and the user experience.
4. When you talk about AI in hearing aids, does it work all the time or only in certain situations?
It should be apparent that in this schema, another way to classify the application of AI within the hearing aid industry is “where” and “when,” as shown in Table 1. AI can be carried out via several devices, including supercomputers operating on data collected in the past as when designing new algorithms or on future data that will be obtained from a multitude of hearing aids and users as when conducting analyses. It can be carried out in the near future on the same data in the cloud, given the time it takes to transmit the information, process it, and send it back to the hearing aids. When carried out on smartphones, presumably, the intent will often be to relay the information back to the hearing aid or user relatively quickly, but not quickly enough to be considered real-time or with a short enough delay to be within the tolerance window for audio-processed signals (<10-30 milliseconds, depending on the severity of loss and listening environment). Finally, if AI were performed in the hearing aid for denoising purposes, it would need to operate in real-time or close to it.
It remains unclear whether today’s hearing aids perform real-time AI computations or merely utilize pre-trained AI solutions. If they do perform AI computations, then they are not like the kind we typically associate with ‘deep learning’ because while the number of parameters in a hearing aid may be in the hundreds, the number in an AI model may be in the millions or billions (Crow et al., 2021). Oh, and do not forget, the more computations there are, the faster the battery will drain; even while we have rechargeable hearing aids, they still need to go a full day on a single charge. In time, dedicated chips for performing AI will be smaller, faster, and more energy efficient, so we will eventually witness more and more being done inside the hearing aid.
Table 1. Categorization for the application of AI in the hearing aid industry based on “where” and “when” AI operations occur. The left column lists the devices used for AI processes, including supercomputers for designing algorithms with historical data and smartphones for quicker data relay to users or hearing aids, albeit not in real-time. The right column specifies the timing of AI operations, such as delayed processing in the cloud and real-time processing within hearing aids for denoising purposes.
5. Okay, I’m starting to see the big picture—it's time for some details. Let’s start at the top: How is AI used in research and development?
Key milestones in AI development for hearing aids span the introduction of AI-trained environmental classifiers in the 2000s (e.g., Schum, 2004; Schum and Beck, 2006) to recent advancements in AI-trained noise reduction algorithms and AI-driven methods for personalizing hearing aid sound settings in nuanced situations. Following the advent of digital hearing aids with an increasing number of programmable features, engineers probably realized that manual adjustments by a user would be impractical due to the limited user interfaces available at the time (before the era of smartphones). Moreover, even if users could adjust these settings, coordinating them to match their listening intentions, needs, or preferences across varying situations would be physically challenging, cognitively demanding, and ultimately unreliable (e.g., Jensen et al., 2019). Also, the speed required to adjust to dynamic listening environments is beyond manual capabilities. This necessitated the development of automated systems based on environmental classifiers. Just like a modern camera can automatically adjust its settings to capture the best photo in different lighting conditions, these systems in hearing aids adjust sound settings to provide the best listening experience in various environments. However, these classifiers needed a minimum level of accuracy and reliability, as errors could lead to user dissatisfaction, just like a photo that is too light or dark or one that blurs a beautiful background or incorrectly focuses on the wrong subject, ruining the intended effect.
In designing these classifiers, one approach was for engineers to establish predictive rules based on their assumptions about different environments, such as overall level, signal-to-noise ratio, and sound source type and locations (Schum, 2004). This method is constrained by knowledge limitations, the engineers’ expertise, and the human inability to fully understand the complex and often hidden relationships among various acoustic features that could reliably classify the listening environment (A.T. Bertelsen in Strom, 2023). An alternative approach, adopted early by some manufacturers, involved machine learning to train classifiers on large datasets of acoustic signals that a panel of human listeners had previously categorized (e.g., Hayes, 2021).
6. I’ve been fitting hearing aids with classifiers for my entire career. How has the development of these tools progressed in the context of modern hearing aids; are they all the same?
Some users may worry about the complexity of AI-enhanced hearing aids. However, they are designed to be user-friendly, with intuitive controls and automatic adjustments that minimize the need for manual intervention. Ideally, hearing aids should be as unobtrusive as glasses—worn but unnoticed. AI-driven hearing aids continuously classify sounds and select the optimal acoustic parameters for each situation, adapting to various soundscapes such as quiet rooms, noisy restaurants, or crowded streets and adjusting settings to suit each specific environment. The accuracy of these classifiers in particular settings significantly influences a user’s hearing experience (Hayes, 2021). Users are frequently reminded of their hearing loss when they have to manually adjust settings or tolerate unsuitable sound levels because necessary adjustments cannot be made. This underscores the importance of automatic environment classification in hearing aids, which can significantly enhance the user experience by reducing the need for manual intervention.
As Hayes (2021) emphasizes, precise classification is crucial for the effective performance of modern hearing aids. The success of the initial fitting in the clinic depends heavily on the accuracy of the hearing aid’s classifier. If the classifier incorrectly identifies the acoustic environment — such as mistakenly detecting a music setting when the user is in a quiet conversational setting — the hearing aid will perform suboptimally because it is optimized for the incorrect scenario.
Modern hearing aids possess significantly more processing power than earlier models, allowing them to integrate a broad range of acoustic features during the classification phase. This capability is enhanced by inputs from the microphones of bilateral hearing aids and other sensors that provide detailed information about the locations of sound sources and the user’s interactions within their environment. Advanced AI techniques utilize these inputs, managing complex and expansive datasets of acoustic scenes to dynamically adapt to changing environments without user intervention. For example, one manufacturer has recently claimed that their latest generation of hearing aids makes “80 million sound analyses and automatic optimizations every hour [one every 15 microseconds] … nearly two billion adjustments in a day” (A. Bhowmik as quoted in Sygrove, 2023).
Despite these advancements, modern classifiers are not perfect. According to research by Hayes et al. (2021), while hearing aid classifiers from major brands generally align when categorizing simple environments, discrepancies emerge in more complex situations. There are noticeable differences in how various manufacturers develop and implement them, influenced by the quantity and quality of the training datasets, as well as the underlying philosophical approaches to classification. For example, imagine several chefs are given the same ingredients to make a dish, but each chef has a different cooking philosophy. One chef might prioritize bold flavors and use more spices, while another might focus on the texture and keep the seasoning light. Or imagine several artists are asked to paint the same landscape, each with a unique style and focus. One artist might highlight the vibrant colors of the flowers, while another emphasizes the shapes of the trees, and yet another concentrates on the interplay of light and shadows.
Similarly, each manufacturer’s hearing aid classifier focuses on different aspects of the listening environment based on their design choices. In a noisy environment with speech, one classifier might prioritize reducing overall noise while another enhances the clarity of the speech. These differences in focus become especially noticeable in complex acoustic scenes, where the subtle details and variations require more sophisticated and subjective interpretation, just as chefs might diverge more in their approaches when preparing a complex dish and as artists’ individual styles become more apparent in intricate landscapes.
7. Once the classifier has done its job, how can AI be used to improve speech understanding?
AI is also revolutionizing how hearing aids process signals to enhance speech understanding and comfort in noisy environments. One manufacturer has integrated AI with directional noise reduction techniques to develop a sophisticated, AI-trained chip that enhances traditional noise reduction methods. Andersen et al. (2021) describe a deep learning-based noise reduction algorithm. Unlike simpler models, this deep neural network was trained on a diverse database of noisy and clean speech examples captured from real-world environments with a spherical array of 32 microphones. Essentially, the directional system in the hearing aid is exploited to derive a more accurate estimate of SNR, which is then fed to the onboard, AI-trained chip that attenuates time-frequency snippets with unfavorable SNR and amplifies those with favorable SNR. This is possible because one directional pattern can be presented to the user while the hearing aid simultaneously evaluates other directional patterns for use by other algorithms (see also Jespersen et al., 2021). The increased accuracy of the SNR estimates provided by the integrated system combined with AI can increase speech intelligibility, unlike conventional single-channel noise reduction techniques (Andersen et al., 2021).
8. I have seen advertisements from a few manufacturers making a big deal about “intent.” What is all this about?
While AI-driven noise reduction and environment classification can significantly enhance the hearing aid user experience by automatically adjusting settings to suit different acoustic environments, they sometimes fail. To understand how a hearing aid with perfect classification and speech segregation can fail to satisfy a user, consider that two individuals with the same hearing loss in the same acoustic environment can have different listening needs, preferences, or intent. Or, more plausibly, consider that the same person in the same acoustic environment can have different listening needs, preferences, or intent depending on the context or time of day (Beck and Pontoppidan, 2019).
Figure 5. ChatGPT illustration of a noisy restaurant, a challenging setting for those with hearing loss. It shows a waiter and an older gentleman in a blue suit jacket, both with the same hearing loss and hearing aid model but different auditory needs. The patron benefits from directional settings like beamforming to focus on his companion, while the waiter requires an omnidirectional setting for awareness of his surroundings and customers, highlighting the need for adaptable hearing aid technologies.
Figure 5 shows a typical busy and noisy restaurant — a difficult listening environment even for a person with normal hearing, especially for someone with hearing loss. Assume that the waiter and the older gentleman in the blue suit jacket sitting behind him have the same hearing loss and hearing aid model. Should the hearing aid behave the same for the waiter and the patron? No, of course not. The obvious solution for the patron would be some form of directionality (there are a handful of configurations on the market), even beamforming (focused directionality), so he can converse with his dinner companion. However, the signal processing chosen for the patron would be detrimental to the waiter, who needs to have situational awareness provided by an omnidirectional setting.
In the scenario above, traditional acoustic information is insufficient to distinguish how users intend to use their hearing in a given listening environment. As depicted in Figure 6, other listening intents include switching between conversations, as when extended families get together during the holidays; eavesdropping on conversations to the side and behind the user; listening to other sound sources like music playing in the background or birds at the park; or simply wanting to tune out the world in favor of listening comfort. This limited sample lets you understand how two individuals in the same acoustic environment might want to listen differently.
Figure 6. Depictions demonstrating how traditional acoustic data might not fully capture individual listening intents in various environments, from left-to-right to top-to-bottom: switching conversations at a family gathering, eavesdropping, enjoying background music, desiring solitude, and listening to nature sounds. This emphasizes the need for hearing aids to adapt to diverse personal listening preferences even within the same environment.
Individuals with normal hearing utilize different acoustic cues to segregate and selectively focus on various sound sources that reach their ears. This task can become increasingly challenging in environments where sounds are complex and blurred by reverberation. For people with sensorineural hearing loss, this difficulty is compounded due to their reduced ability to detect these subtle acoustic cues essential for identifying and distinguishing between sound sources (Grimault et al., 2001; Rose and Moore, 2005; Shinn-Cunningham and Best, 2008). Consequently, merely amplifying sounds may not be enough for those with hearing loss, as it does not address the core issue of sound source segregation. In some cases, traditional hearing aid processing may actually hinder segregation (Beck, 2021).
9. So, how does AI help manage these complex listening situations?
A practical solution for hearing aids involves automatic adjustments like bilateral directionality, unilateral directionality, and beamforming, which are applied based on the acoustic environment (Jespersen et al., 2021). For instance, if a classifier detects speech from the front with background noise, it may activate a directional setting. Conversely, omnidirectionality is chosen to enhance environmental awareness if speech predominates from the sides or behind, or if the user is moving.
Sensors play a crucial role in enhancing the functionality of hearing aids beyond sound amplification. While microphones are typically known for capturing audio signals for amplification, they also inform the hearing aid’s classifier about the listening environment. This dual function enables more precise adjustments and enhances overall performance. A single microphone can offer basic details such as the overall sound level and the signal-to-noise ratio. As indicated, dual microphones can provide location information about the direction of speech and noise sources while simultaneously presenting the user with a completely different directional response, including omnidirectionality (Jespersen et al., 2021). This allows the hearing aid to be responsive to the user’s dynamic environment, toggling between focused listening modes and omnidirectionality to suit different listening needs or intents.
Moreover, an increasing number of hearing aids are incorporating motion sensors, such as accelerometers, gyroscopes, and magnetometers. Branda and Wurzbacher (2021) explain how sensors can determine if the user is stationary or in motion, adjusting the hearing aid’s directional settings accordingly. For example, a stationary user in a noisy environment (e.g., the blue-suited patron in the restaurant in Figure 6) might benefit from directional features, while a moving user (e.g., the waiter navigating through the restaurant in Figure 6) might require omnidirectional settings to stay aware of his surroundings.
Additionally, it is important to consider the classifier’s ability to detect user movement in conjunction with speech sources. For instance, an omnidirectional response is typically most effective if the classifier identifies that the user is moving while a single speech source is present. This is because people are often positioned side-by-side or with one slightly behind the other during activities such as walking and talking. With all the range of motions associated with different types of movement — encompassing activities like walking, jogging, bicycling, driving, or even more abrupt movements such as falling — optimal sensitivity and specificity would come from an AI-based solution (e.g., Fabry and Bhowmik, 2021).
10. What can be done about more complex environments with more than one talker, like the large family gathering you mentioned?
Recently, one manufacturer has added head movements to its arsenal of sensors. The idea is that a hearing aid user’s role or engagement in a listening environment can be better classified by combining head movements with information provided about the user’s body movements, the location of different sound sources, and the presence of conversation (i.e., back and forth dialog between the user and one or more others).
As Higgins et al. (2023) explain, a rich set of information can be gathered by the three axes of rotation associated with head movement, especially when considered in the context of social and cultural norms. Higgins et al. explain that head movements are more important than you might think in conversations between two or more people. When someone is speaking, they often use head movements like nodding or shaking their head to highlight their points or even take charge of the conversation. For example, quick nods might be used to interrupt someone or show strong agreement. Listeners, on the other hand, use their head movements differently. They might tilt their head or lean in closer to hear better, especially if it is noisy. They also tend to mimic the speaker’s head movements, which helps them connect better and show that they are really listening.
Head movements also give clues to anyone watching the conversation (Higgins et al., 2023). They can help someone determine if the people talking know each other well, like distinguishing between friends chatting and strangers talking. Also, how people move their heads can show if they are feeling strong emotions or even if they might be trying to deceive the other person. For instance, more head nodding and mirroring can happen when someone is not being sincere.
At large family gatherings (e.g., like the one shown at the top of Figure 6), where multiple conversations and background noise co-occur, hearing aids can theoretically benefit from understanding head movements. By recognizing these patterns, hearing aids can enhance the user’s ability to focus and engage in conversations, thereby dynamically adjusting to the user’s listening intent. For instance, if a hearing aid detects that the user is turning their head frequently towards different speakers, it could adjust its parameters to prioritize sounds coming from the direction the user is facing by applying speech enhancement algorithms and/or increased gain for voices directly ahead while reducing background noise from other directions. Similarly, if the hearing aid picks up on the user nodding or tilting their head in response to someone speaking from a specific direction, it could momentarily enhance the sound from that area to improve clarity and understanding. Moreover, if the hearing aid uses AI to detect patterns like head tilting or leaning in, which might indicate an attempt to hear better in noisy environments, it could automatically switch to a setting better suited for noisy environments, making it easier for the user to follow conversations.
Directional microphones are designed to enhance speech understanding by focusing on sounds originating from a specific direction, typically in front of the user. However, this directional focus can sometimes interfere with the user’s ability to quickly and accurately localize sounds coming from new or different directions, especially if these are at large angles from the initial focus (Higgins et al., 2023). This limitation can be particularly noticeable when a user needs to turn their head to engage with a new target of interest, such as a person speaking from the side or behind. To address this issue, hearing aids could be designed to temporarily switch between directional modes based on the user’s head movements. When the hearing aids detect that the user is turning their head significantly, suggesting they are searching for a new sound source, they could momentarily adopt a more omnidirectional microphone setting to allow the user to orientate to the thing they want to hear. Once the new target of interest is identified and the user’s head is more stable, the hearing aids could switch back to a directional mode to allow the user to selectively attend to the desired sound source.
11. If head movements are so informative, surely eye movements must also carry a lot of information. Can eye movements be used to help hearing aid processing?
Integrating eye gaze to guide the directionality of hearing aids is a promising research area that could influence how hearing aids function in complex environments (Kidd, 2017; Roverud et al., 2017; Culling et al., 2023). Using eye gaze to control directional settings could create a more intuitive and responsive user experience, as people typically look toward the source of sound or interest. However, Higgins et al. (2023) note that implementing this feature faces several challenges. The correlation between eye and head movements can vary significantly depending on the specific activity and the surrounding environment, making it difficult to create a one-size-fits-all solution. Additionally, accurately tracking eye movements in a device as small and unobtrusive as a hearing aid requires sophisticated technology to handle significant amounts of data and perform real-time processing.
Advancements in microchip technology and AI might eventually overcome these challenges. As microchips become more powerful and capable of processing larger datasets more efficiently, AI algorithms could be developed to intelligently integrate data from eye and head movements. This would allow the hearing aids to more accurately predict and respond to a user’s focus of attention, adjusting the directionality of microphones to enhance the sounds of interest. Moreover, future AI models could learn from individual user behaviors, adapting to each user’s unique eye and head movement patterns in different situations.
12. With all these sensors, can I ditch my Fitbit?
Advances in AI technologies, such as those seen in voice recognition and wearable devices, are influencing the development of hearing aids. As detailed by Fabry and Bhowmik (2021), these cross-industry innovations are revolutionizing hearing aids, turning them into versatile devices. Some hearing aids now offer comprehensive health and wellness monitoring by continuously tracking physical activities and social interactions. With embedded sensors and AI, one manufacturer’s hearing aids can detect falls and automatically alert designated contacts (Fabry and Bhowmik, 2021). As these authors note, studies indicate that ear-worn fall detection systems often outperform traditional neck-worn devices in terms of sensitivity and specificity during simulated falls (Burwinkel et al., 2020). Additionally, data suggests that hearing aids are more accurate than wrist-worn activity trackers in counting daily steps (Rahme et al., 2021).
Furthermore, smartphone apps can incorporate social engagement metrics by tracking daily usage hours, time spent in speech-rich environments, and the variety of listening situations encountered to encourage users to wear their hearing aids more regularly and participate in conversations (Fabry and Bhowmik, 2021). Thus, tools provided by apps can act as a “hearing health coach” that promotes consistent hearing aid use, similar to how a personal trainer adjusts workout routines based on their client’s progress and feedback. They can also provide audiologists with valuable data to optimize the devices for various situations.
13. How else can “smart” phones make hearing aids smarter?
Connecting hearing aids to digital assistants and smartphone technology significantly enhances their capabilities, giving them an added layer of intelligence. Considering that some hearing aids can function similarly to wireless earbuds — the possibilities multiply exponentially. By integrating AI-driven virtual assistants or conversational interfaces into hearing aid apps or companion devices, users can control and adjust their hearing aids using simple voice commands. This makes managing settings and preferences more intuitive and user-friendly. Furthermore, connecting to the cloud via a smartphone allows hearing aids to access advanced features, such as real-time language translation, with only a slight delay. This capability can particularly benefit travelers or individuals living in multilingual communities.
Another example, described by Fabry and Browmik (2021), employs the smartphone microphone as a remote microphone and the smartphone technology as an off-board AI processor to enhance speech intelligibility in listening environments with unfavorable signal-to-noise ratios, particularly for users with more severe hearing loss who have a higher tolerance for delays associated with signal processing and transmission.
14. What about when the hearing aids do not act so smart because the classifier makes a mistake or the automatic solution is wrong?
Circling back to Figure 4, where this in-depth conversation started, AI can help when automatics fail to accurately capture the user’s listening need, intent, or preference. AI algorithms — whether in a smartphone, the cloud, or integrated into the hearing aid — can enhance the user experience by adapting to their unique situations. The algorithms can learn from users’ interactions with their hearing aids and the manufacturer’s app to customize sound settings for specific environments. They can also monitor and analyze the user’s usage patterns, such as time spent in different acoustic environments or satisfaction ratings. The results of this analysis could then be transmitted to the hearing aids (Bramsløw and Beck, 2021), thereby adapting their behavior and responses without requiring a visit to the audiologist for reprogramming (Jensen et al., 2023), much like how a streaming service recommends movies based on your viewing history; by understanding your preferences, these services suggest content that you are more likely to enjoy. For less tech-savvy users and less technologically advanced hearing aids, the information gained from AI analysis can help the audiologist fine-tune the settings and/or create special programs for those times and places automatics fail.
I will use two cases to illustrate how these algorithms can help override the automatics and provide the user with a personalized solution. The first, as explained by Fabry and Browmik (2021), when faced with a challenging listening environment, a hearing aid user can easily initiate assistance using a simple interface like a double-tap or push-button. Upon activation, the hearing aid captures an “acoustic snapshot” of the environment and optimizes speech intelligibility by adjusting parameters across eight proprietary classifications, ranging from quiet to noisy environments. Some parameter adjustments include modifications to gain, noise management, directional microphone settings, and wind noise management. Importantly, this process does not require smartphone or cloud connectivity since all the processing uses the onboard, AI-trained algorithm.
Balling et al. (2021) also explain how customized solutions for specific listening environments can be achieved through simple gain adjustments across three frequency channels. However, implementing these adjustments would require an extensive number of comparisons. To address this, the core of their technology employs AI to iteratively refine a series of A-B comparisons, enabling an optimal solution to be found often with 20 or fewer comparisons. Users who find themselves in a situation where the automatics fail to capture their listening need, intent, or preference can activate an app on their smartphone, which interfaces with their hearing aids. This technology is particularly efficient because it captures the degree of preference for one setting over another, providing the AI algorithm with a continuous range of values rather than a limited set of discrete responses. This continuous feedback allows the algorithm to more accurately and quickly identify the preferred parameter settings for the user, which can be used to train the automatics for a personalized experience the next time the user is in a similar situation. If a more generalized solution is appropriate, this information can be shared with the audiologist, who can make the necessary programming changes.
15. There must be a goldmine of data with all the potential information sent by the hearing aids and user interactions to the cloud. How do the manufacturers use this information?
This question brings us to our final stop along the path in Figure 4 — Analysis. First, I should acknowledge that data security is a priority in today’s contradictory world, where some people have a strange fascination with sharing the intimate details of their daily lives on social media yet wish to remain anonymous to anyone who is not their “friend.” If, indeed, a given manufacturer is storing limited and anonymous data in the cloud, users must consent to this. According to one manufacturer (Balling et al.,2021), these data include information on the settings and usage of created programs, the activities and intentions indicated by users when the algorithm was activated, and the settings compared along with the associated degree of preference. The manufacturer can leverage this anonymized data to crowdsource improvements for the AI algorithm. Analyzing patterns from multiple users makes the algorithm more efficient, requiring fewer comparisons to find the optimal solution for each user. This process benefits both current and future users as more optimal solutions are sent back to the hearing aids or the smartphone interface. As data, AI technology, and computing power advance, we can expect even smarter, more adaptive hearing aids that continuously improve based on user feedback and usage patterns.
The hearing aid industry leverages AI to analyze vast amounts of data—such as user preferences, behaviors, and environmental conditions—to identify patterns and make predictions for future research and development. For example, Balling et al. (2021) illustrate how AI was used to process extensive user comparison data, summarizing it to visualize trends. Specifically, AI tracked how users adjusted their hearing aids in various settings, including noisy restaurants, quiet rooms, or activities like watching TV and attending parties. This demonstrates AI’s capability to uncover patterns and correlations that might not be immediately apparent to humans. Importantly, AI predictions are dynamic; they can be continuously refined with additional data. As user preferences evolve with new listening environments or advancements in hearing aid technology, AI algorithms adapt to provide increasingly accurate and personalized recommendations.
Finally, Burwinkel et al. (2024) demonstrated how speech-to-text recognition can be utilized to evaluate algorithm effectiveness by predicting human performance. By analyzing audio recordings from hearing aids in various real-world and controlled environments using the Google speech-to-text recognizer, they compared transcription accuracy and confidence levels to the performance of hard-of-hearing individuals. This comparison allowed them to estimate how well humans would understand speech under similar conditions. Their findings showed that a speech-to-text recognizer can be a reliable predictor of human speech intelligibility with hearing loops and telecoil inputs. This example highlights how AI can accelerate hearing aid algorithm development by enabling rapid, cost-effective testing of new algorithms. Using speech-to-text systems, hearing aid engineers can quickly assess speech intelligibility and effectiveness under various conditions, reducing the need for extensive human trials and providing immediate, objective feedback.
16. Wow! It seems that AI is being used everywhere in hearing aid design. What else could be left for it to do?
Interestingly, AI has also been used for quality control, improving reliability, preventing unexpected performance degradations, and extending overall lifespan (of the hearing aid, not the person). Similar to its use in manufacturing for improved quality control and anticipating equipment failures, AI can address potential issues within hearing aids. For instance, one manufacturer utilizes AI to analyze real-time data collected from hearing aids (Thielen, 2024). This data includes streaming stability, battery performance, hardware integrity, and more. Analyzing this data allows the manufacturer to detect and address problems before they emerge or become more significant; for example, detecting anomalies like sudden battery drain experienced by multiple users. Furthermore, AI can analyze long-term data trends to predict future maintenance needs before they become more significant problems.
17. What could AI advancements mean for the future of hearing aids?
Looking ahead, the potential for AI in hearing aids is vast. From advanced sensors for more precise sound source segregation and integrated head and eye movement for selective attention, the future promises even greater enhancements in hearing aid technology and user experience. Interdisciplinary collaboration between audiologists, computer scientists, and engineers will likely drive major advancements in hearing aid technology. For example, with a recent collaboration between manufacturers like Cochlear, research institutions like the NAL, and tech companies like Google (Aquino, 2023), more rapid advancements in cochlear implant and hearing aid technology may emerge as they leverage diverse expertise to develop cutting-edge solutions that enhance functionality and user experience.
Inspired by the recent advances in AI, we can begin to envision possibilities for hearing aid technology that we could have never dreamt of back in the 1990s when digital hearing aids first came to the market. Future research in AI for hearing aids could focus on areas such as integrating brain-computer interfaces, improving real-time processing capabilities, and enhancing personalized sound environments. Imagine a hearing aid equipped with a microphone front-end composed of thousands of tiny strands, each tuned to a distinct frequency (Lenk et al., 2023). This setup, mimicking a digital cochlea, could precisely separate and analyze various sounds from complex environments filled with overlapping voices and noises (e.g., Wilson et al., 2022; Lesica et al., 2023). Such hearing aids could leverage EEG signals gathered from miniature electrodes within the ear canal to predict and focus on specific auditory inputs the user is most interested in (Lesica et al., 2023; Hjortkjær et al., 2024; Hosseini et al., 2024). Alternatively, a more imminent scenario might involve cloud-based AI systems. These systems could be trained to recognize specific voices and, upon learning, transmit tailored algorithms back to the hearing aid (Bramsløw and Beck, 2021). This would enable users to isolate selected voices from a crowd, enhancing speech understanding in challenging listening environments.
18. As hearing aids become more complex and capable with AI, is there a risk that audiologists might be less involved in the tuning process?
Considering that many audiologists typically leave their patients’ hearing aid settings on ‘default’ or ‘recommended’ due to a fear of compromising a seemingly adequate fitting, and given the increasing complexity of settings with each new iteration of hearing aids, AI will likely be essential for individualizing and optimizing each patient’s device.
As the hearing care industry rapidly evolves with technological advancements, there is a real possibility that competitive pressures may push audiologists out of the fine-tuning process for hearing aids. AI assistants can accompany the user continuously, constantly capturing and adapting to the precise acoustic environment (e.g., Høydal et al., 2020). This technology can discern both immediate needs and long-term adjustments, providing responsiveness and ‘expertise’ (collective information from millions of user interactions) that traditional audiologist appointments cannot achieve (Jensen et al., 2023). Unaware that this future might not be far off, many professionals might assume that these changes will only materialize after retirement or believe that their current patients will not adopt such technology. However, just as the public has gradually embraced rechargeable hearing aids as the technology improved and daily charging became routine, they might also come to accept these advanced features (Høydal et al. 2021; Jensen et al., 2023). Such a shift could fundamentally change how hearing care is delivered, potentially diminishing the audiologist’s role in personalizing hearing aids.
From an industry standpoint, this scenario represents a straightforward opportunity compared to the ambition of developing more sophisticated capabilities, such as flawless speech recognition in noise, distinguishing between multiple speakers, and using brain monitoring technology to focus on a specific speaker while also managing other information streams.
AI-driven, self-tuning technologies, which have been around for at least five years, are becoming increasingly sophisticated and normalized as consumers gradually become more open to adopting them. Taylor and Jensen (2023a) identify the five most common types of service and support during a typical follow-up appointment: fine-tuning, fit-related adjustments, coaching or counseling, feature activation, and service/repair/troubleshooting. To varying degrees, just about all of these could be facilitated by an AI-hearing health assistant or coach.
19. This all sounds ominous; can it get worse?
Combine the precision of AI in individualizing hearing aid fittings with the emergence of over-the-counter (OTC) hearing aids, and it is easy to imagine a new way for consumers to access hearing care. Picture a customer (who is no longer a patient or client) walking into their local big box store and entering a comfortable, well-lit kiosk for an AI-controlled, bonafide audiogram. Upon completion, a large monitor could present them with various hearing aids at different price points, complete with customer reviews. After choosing a pair, an AI system would guide them to insert a probe-microphone tube into their ear canal. The system would instruct them to stop just a few millimeters from the eardrum. Next, they would insert their new hearing aids and press a button to initiate an automatic real-ear measurement (auto REM) that fits to prescriptive targets more accurately than any human could. An automatic acclimatization management system would then adjust based on the customer’s selection of sound examples. While not needing to be perfect initially, an AI hearing coach would continuously fine-tune and counsel them throughout the fitting process over the ensuing days and weeks.
20. Yikes! Where does “HatGPT” leave me?
Taylor and Jensen (2023b) provided some recent thoughts on this very topic. As I remind my students, remaining engaged and proactive in the hearing aid fitting process is crucial. Emphasize the value of patient interactions and adhere to best practices. Make it a priority to stay informed about the latest technologies, even from manufacturers you do not typically use. Being adaptable and ready to embrace change is essential in a field where technology rapidly evolves. By doing so, you safeguard your indispensable role as a professional, ensuring that you enhance, rather than be replaced by, the “machine.”
This is where HatGPT (Hearing Aid Technology Guided by Predictive Techniques) comes into play. HatGPT represents the cutting-edge of AI-driven advancements in hearing aid technology. It is vital to understand and integrate these predictive techniques into your practice. While HatGPT can offer incredible enhancements in fitting precision and patient customization, it cannot replace the unique expertise and human touch that you provide. Your deep understanding of patient needs, ability to empathize, and personalized care are irreplaceable components that no technology can fully replicate. Embrace HatGPT as a tool to augment your practice, ensuring that you remain at the forefront of hearing aid technology and continue to deliver exceptional care.
References
Andersen, A. H., Santurette, S., Pedersen, M. S., Alickovic, E., Fiedler, L., Jensen, J., & Behrens, T. (2021). Creating clarity in noisy environments by using deep learning in hearing aids. Seminars in Hearing, 42(3), 260-281. https://doi.org/gtwc7f
Aquino, S. (2023, April 17). Inside Cochlear and Google’s joint quest to use technology to demystify hearing health for all. Forbes. https://www.forbes.com/sites/stevenaquino/2023/04/17/inside-cochlear-and-googles-joint-quest-to-use-technology-to-demystify-hearing-health-for-all
Balling, L. W., Mølgaard, L. L., Townend, O., & Nielsen, J. B. B. (2021). The collaboration between hearing aid users and artificial intelligence to optimize sound. Seminars in Hearing, 42(3), 282-294. https://doi.org/nb2s
Beck, D. L. (2021). Hearing, listening and deep neural networks in hearing aids. Journal of Otolaryngology-ENT Research, 13(1), 5-8. https://doi.org/gmkdkx
Beck, D. L., & Pontoppidan, N. H. (2019, May 22). Predicting the intent of a hearing aid wearer. Hearing Review. https://hearingreview.com/hearing-products/hearing-aids/apps/predicting-intent-hearing-aid-wearer
Beck, D. L., & Schum, D. J. (2004). Envirograms and artificial intelligence. AudiologyOnline, Article 1082. www.audiologyonline.com
Bramsløw, L., & Beck, D. L. (2021, January 15). Deep neural networks in hearing devices. Hearing Review. https://hearingreview.com/hearing-products/hearing-aids/deep-neural-networks
Branda, E., & Wurzbacher, T. (2021). Motion sensors in automatic steering of hearing aids. Seminars in Hearing, 42(3), 237-247. https://doi.org/nb2t
Burwinkel, J. R., Xu, B., & Crukley, J. (2020). Preliminary examination of the accuracy of a fall detection device embedded into hearing instruments. Journal of the American Academy of Audiology, 31(6), 393-403.
Burwinkel, J. R., Barret, R. E., Marquardt, D., George, E., & Jensen, K. K. (2024). How hearing loops and induction coils improve SNR in public spaces. Hearing Review, 31(2), 22-25.
Chalupper, J., Junius, D., & Powers, T. (2009). Algorithm lets users train aid to optimize compression, frequency shape, and gain. The Hearing Journal, 62(8), 26-33. https://doi.org/nb2v
Crow, D., Linden, J., & Thelander Bertelsen, A. (2021). Redefining hearing aids. Whisper. https://www.whisper.ai/resources/whitepapers/redefining-hearing-aids
Culling, J. F., D’Olne, E. F. C., Davies, B. D., Powell, N., & Naylor, P. A. (2023). Practical utility of a head-mounted gaze-directed beamforming system. Journal of the Acoustical Society of America, 154(6), 3760-3768. https://doi.org/10.1121/10.0023961
Dillon, H. (1999). NAL-NL1: A new procedure for fitting non-linear hearing aids. The Hearing Journal, 52(4), 10-16.
Fabry, D. A., & Bhowmik, A. K. (2021). Improving speech understanding and monitoring health with hearing aids using artificial intelligence and embedded sensors. Seminars in Hearing, 42(3), 295-308.
Grimault, N., Micheyl, C., Carlyon, R., Arthaud, P., & Collet, L. (2001). Perceptual auditory stream segregation of sequences of complex sounds in subjects with normal and impaired hearing. British Journal of Audiology, 35(3), 173-182.
Hayes, D. (2021). Environmental classification in hearing aids. Seminars in Hearing, 42(3), 186-205.
Higgins, N. C., Pupo, D. A., Ozmeral, E. J., & Eddins, D. A. (2023). Head movement and its relation to hearing. Frontiers in Psychology, 14, Article 1183303.
Hjortkjær, J., Wong, D. D. E., Catania, A., Märcher-Rørsted, J., Ceolini, E., Fuglsang, S. A., Kiselev, I., Di Liberto, G., Liu, S. C., Dau, T., Slaney, M., & de Cheveigné, A. (2024). Real-time control of a hearing instrument with EEG-based attention decoding. bioRxiv.
Høydal, E. H., Fischer, R.-L., Wolf, V., Branda, E., & Aubreville, M. (2020, July 15). Empowering the wearer: AI-based Signia Assistant allows individualized hearing care. Hearing Review, 27(7), 22-26.
Høydal, E. H., Jensen, N. S., Fischer, R.-L., Haag, S., & Taylor, B. (2021). AI assistant improves both wearer outcomes and clinical efficiency. Hearing Review, 28(11), 24-26.
Hosseini, M., Celotti, L., & Plourde, E. (2024). Speaker-independent speech enhancement with brain signals. IEEE Transactions on Neural Systems and Rehabilitation Engineering.
Jensen, N. S., Hau, O., Nielsen, J. B. B., Nielsen, T. B., & Legarth, S. V. (2019). Perceptual effects of adjusting hearing-aid gain by means of a machine-learning approach based on individual user preference. Trends in Hearing, 23, 1-23.
Jensen, N. S., Taylor, B., & Müller, M. (2023). AI-based fine-tuning: How Signia Assistant improves wearer acceptance rates. AudiologyOnline, Article 28555. www.audiologyonline.com
Jespersen, C. T., Kirkwood, B. C., & Groth, J. (2021). Increasing the effectiveness of hearing aid directional microphones. Seminars in Hearing, 42(3), 224-236.
Kidd, G. Jr. (2017). Enhancing auditory selective attention using a visually guided hearing aid. Journal of Speech, Language, and Hearing Research, 60(10), 3027-3038.
Lenk, C., Hövel, P., Ved, K., Durstewitz, S., Meurer, T., Fritsch, T., Männchen, A., Küller, J., Beer, D., Ivanov, T., & Ziegler, M. (2023). Neuromorphic acoustic sensing using an adaptive microelectromechanical cochlea with integrated feedback. Nature Electronics, 6(May), 370-380.
Lesica, N. A., Mehta, N., Manjaly, J. G., Deng, L., Wilson, B. S., & Zeng, F.-G. (2021). Harnessing the power of artificial intelligence to transform hearing healthcare and research. Nature Machine Intelligence, 3(10), 840-849. https://doi.org/10.1038/s42256-021-00394-z
Pouliot, B. (2024, May 7). Fostering social connections: The essential role of speech understanding. Audiology Blog. Retrieved from https://audiologyblog.phonakpro.com/fostering-social-connections-the-essential-role-of-speech-understanding/
Rahme, M., Folkeard, P., & Scollie, S. (2021). Evaluating the accuracy of step tracking and fall detection in the Starkey Livio artificial intelligence hearing aids: A pilot study. American Journal of Audiology, 30(2), 182-189. https://doi.org/10.1044/2021_AJA-20-00156
Rose, M. M., & Moore, B. C. J. (2005). The relationship between stream segregation and frequency discrimination in normally hearing and hearing-impaired subjects. Hearing Research, 204(1-2), 16-28. https://doi.org/10.1016/j.heares.2004.10.010
Roverud, E., Best, V., Mason, C. R., Streeter, T., & Kidd, G. Jr. (2018). Evaluating the performance of a visually guided hearing aid using a dynamic auditory-visual word congruence task. Ear and Hearing, 39(4), 756-769. https://doi.org/10.1097/AUD.0000000000000532
Shinn-Cunningham, B. G., & Best, V. (2008). Selective attention in normal and impaired hearing. Trends in Amplification, 12(4), 283-299. https://doi.org/10.1177/1084713808325306
Schum, D. J. (2004, June 7). Artificial intelligence: The new advanced technology in hearing aids. AudiologyOnline. www.audiologyonline.com
Strom, K. (2023, April 13). Artificial intelligence (AI) and DNN in hearing aids: Getting past all the noise–An interview with Whisper’s Andreas Thelander Bertelsen. Hearing Tracker. https://www.hearingtracker.com/news/artificial-intelligence-ai-and-dnn-in-hearing-aids-interview-with-andreas-thelander-bertelsen
Sygrove, C. (2024, October 24). Hearing aids with artificial intelligence (AI): Review of features, capabilities and models that use AI and machine learning. Hearing Tracker. https://www.hearingtracker.com/resources/ai-in-hearing-aids-a-review-of-brands-and-models
Tanveer, M. A., Skoglund, M. A., Bernhardsson, B., & Alickovic, E. (2024). Deep learning-based auditory attention decoding in listeners with hearing impairment. Journal of Neural Engineering. Advance online publication. https://doi.org/10.1088/1741-2552/ad49d7
Taylor, B. & Jensen, N. S. (2023a). How AI-driven hearing aid features and fresh approaches to counseling can promote better outcomes: part 1, the catch-22 of follow-up care. AudiologyOnline, Article 28670. www.audiologyonline.com
Taylor, B. & Jensen, N. S. (2023b). How AI-driven hearing aid features and fresh approaches to counseling can promote better outcomes: part 2, towards more empowered follow-up care. AudiologyOnline, Article 28727. www.audiologyonline.com
Taylor, B., & Mueller, H. G. (2023). Research QuickTakes Volume 2: identification and management of post-fitting hearing aid issues. AudiologyOnline, Article 28676. www.audiologyonline.com
Thielen, A. (2024). Leveraging machine learning and big data to enhance the customer experience. Audiology Blog. Retrieved from https://audiologyblog.phonakpro.com/leveraging-machine-learning-and-big-data-to-enhance-the-customer-experience/
Wilson, B. S., Tucci, D. L., Moses, D. A., Chang, E. F., Young, N. M., Zeng, F. G., ... & Francis, H. W. (2022). Harnessing the power of artificial intelligence in otolaryngology and the communication sciences. Journal of the Association for Research in Otolaryngology. https://doi.org/10.1007/s10162-022-00846-2
Citation
Alexander, J. (2024). 20Q: Artificial intelligence in hearing aids - HatGPT. AudiologyOnline, Article 28996. www.audiologyonline.com